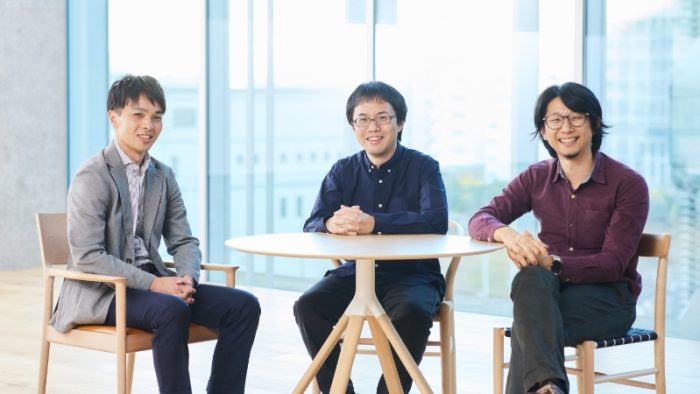
We came to know about Kaggle as we sought ways to upgrade our skills
– First, tell us how you came to join Kaggle, as well as your job situation at the time.
Miyatani: I joined Kaggle in 2018. At the time, I was an engineer working on the digital SLR camera α™ (Alpha). I had this idea of introducing deep learning to cameras ahead of the rest of the world and was involved in efforts to improve the image quality of cameras and to develop new algorithms for auto focus among other things. Those days were the developing period of AI where use cases of real-world deep learning application began to emerge and new techniques were announced almost daily. We didn’t have a wide range of learning contents, like we do now, and I was struggling to figure out how I could catch up with this technology and incorporate it into my own skill set. It was in those days that I heard the reputation about Kaggle being a good place to learn about AI. So, I started to take part in Kaggle competitions outside working hours.
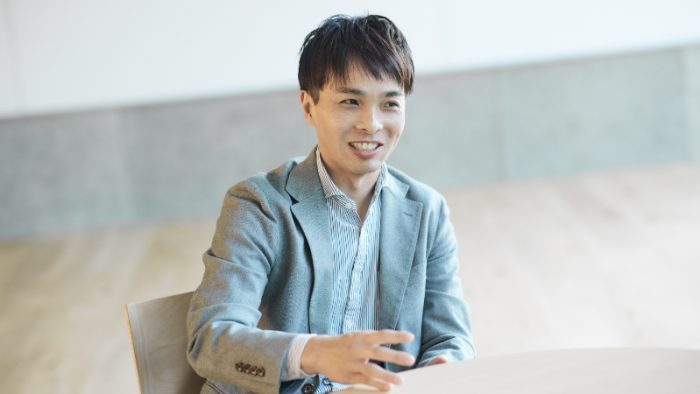
Yoshitaka Miyatani: When he joined Kaggle, Mr. Miyatani belonged to the Digital Imaging Group, Sony Imaging Products & Solutions Inc. Currently, he works for Sony Semiconductor Solutions Corporation and is in a managerial position overseeing departments in charge of the development of AI for new sensing image sensors.
Kobayashi: I joined Kaggle to upgrade my skills, too. Back then, I was an engineer in the semiconductor business domain and assigned to develop AI technology for estimating the positions of human bodies and hand joints, as well as a touchless UI that allows you to operate a display without touching it by utilizing the AI technology. For the first time in my life, I stepped into the world of AI. I somehow managed to accomplish the assigned technology development tasks with the cooperation of R&D experts but, at the same time, I really felt my lack of skills and knowledge. I realized that the technology I had developed could not estimate the positions of objects other than joints and that there was so much more I had to understand about AI. This motivated me to build up experience on AI from scratch, and I joined Kaggle in 2021.
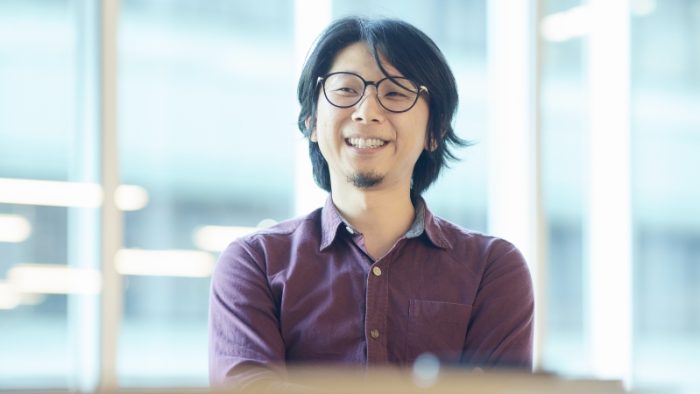
Suguru Kobayashi: When he joined Kaggle, Mr. Kobayashi belonged to the Imaging & Sensing Edge Core Technology Division of Sony Semiconductor Solutions Corporation. Currently, he works for the System Software Technology Center of Sony Corporation and is engaged in the development of AI technology and applied technologies for the digital SLR camera α™.
– Mr. Miyahara, did you also take part in Kaggle to upgrade your skills?
Miyahara: I joined Kaggle in 2019 because I simply found it interesting, not because I thought it would help my career. I am a member of an R&D team working on a range of matters from research and development regarding data analysis to social implementation of technologies. Since long ago, I have been thinking about making machine learning, data analysis, and other advanced technologies readily accessible to anyone. I am now the leader of a project on a prediction analysis tool called Prediction One. At the time I joined Kaggle, we handled only small-scale tabular datasets in our work. I was curious to work with larger tabular datasets and other types of data like natural language data and image data, so I decided to take part in Kaggle.
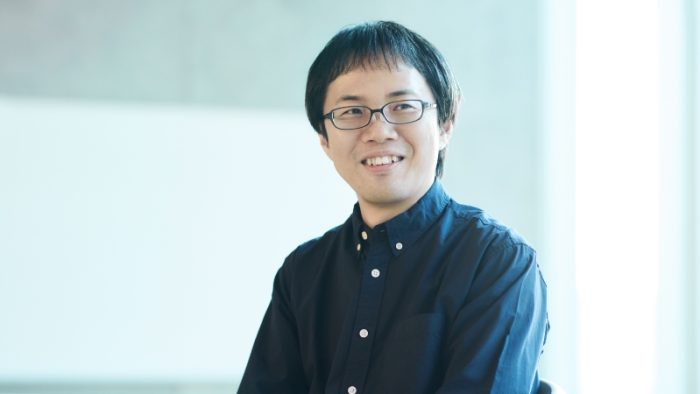
Masanori Miyahara: Since he joined Kaggle until now, Mr. Miyahara has worked for the Data Science and Application Development Department, System Platform Technology Division, Technology Infrastructure Center of Sony Group Corporation.
We increase our value as engineers as we learn while having fun
– Tell us about the competition activities you did at Kaggle. And, how did you get promoted to the Kaggle Master tier?
Miyatani: At the time, I was an engineer working with images. So, I began with competitions on image data. I found this area familiar and thought I would be able to perform well in those competitions. Before long, however, I wanted to broaden my knowledge of AI in general and started to participate actively in competitions on topics like tabular data and mathematical optimization.
A tactic I learned as I entered one competition after another is to increase the amount of input data and accelerate the trial-and-error process. Looking at the solutions of top Kagglers, I was often shocked about the difference between these competitors and me and wondered how they could come up with such ideas. When I asked the Kagglers to find out, they told me they had found the solutions by accident while trying their ideas hundreds, or even thousands, of times. Which made me realize that the only difference between the top Kagglers and me is that they tried while I didn’t. Since then, I have tried every idea that comes to mind if I see any potential in it, no matter how small that potential may be. I have been learning eagerly, improving my skills, and devoting every bit of my spare time to thinking about new ideas. My performance naturally improved in the process, and I got promoted to Kaggle Master.
– “Do Everything” is a phrase that Kagglers use frequently. You put it into practice. Now, what about you, Mr. Kobayashi and Mr. Miyahara?
Kobayashi: At the beginning, I didn’t have much knowledge about AI, so I started by studying the solutions to various competitions. Some of the competition topics were related to what I was doing in my work, and I was impressed with the wealth of diverse approaches presented in the competitions that I could not have imagined. At the same time, I was motivated to explore uncharted territory and became eager to get on the leaderboard.
After that, I spent almost all of my spare time on Kaggle competitions. I chose to participate in higher-level competitions one after another because I thought that competing with talented rivals would boost my skills. During the first year, my efforts got nowhere. I reviewed my approach after every competition, studied top winners’ solutions, and kept absorbing new ideas and techniques. In the process, I started to win medals and ultimately received the title of Kaggle Master. I was honored to receive the title, but what was equally fantastic was the fulfilling process of growth I experienced while I kept making a lot of mistakes.
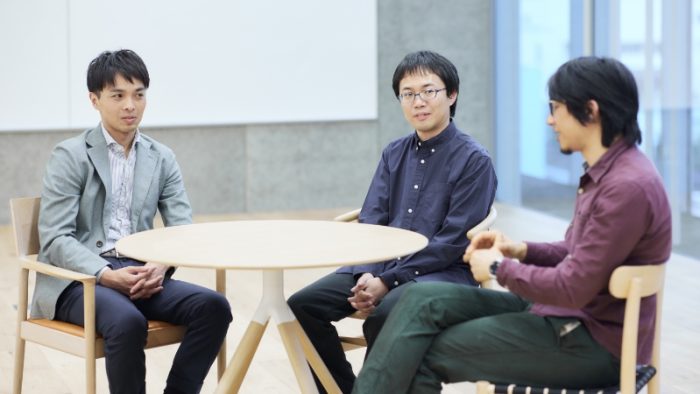
Miyahara: As I said earlier, I kept entering many different competitions in the spare time I had between work and child care, because I wanted to work with various kinds of data. When I actually participated in a Kaggle competition, it fascinated me right away because it had all the fun elements of a game. For example, you can enjoy building your pipeline little by little. You get excited to see your rank jump on the leaderboard, you get nervous just before nine o’clock in the morning on the deadline day of the competition, you get surprised by the shake-up *2 when results are announced, et cetera, et cetera. I was deeply absorbed in these competitions like I was when playing a video game.
At the time, though, I loved my job and I also wanted to take an active role in taking care of my child. So, I was competing on Kaggle as a hobby to make sure it did not affect my job or child-rearing. To be concrete, I first worked very hard at my job and child-rearing and then took part in competitions as a reward for my hard work. I kept entering competitions like this, enthusiastically figuring out a number of solutions each time. And the next thing I knew, I got promoted to Kaggle Master. That’s how I felt. Sometimes, it was hard to strike a balance between work, child-rearing, and Kaggle. But my interest in AI never wears out, and this passion has kept me going.
*2 At Kaggle, only part of the evaluation data was made public during a competition. Since the published data is different from the data used for the final evaluation, participants are required to develop versatile models. This makes it possible that the positions on the leaderboard may change at the end of the competition, which is called a shake-up.
– So, one thing in common is that you all became Kaggle Masters while you enjoyed working on competitions. By the way, what competition or event is the most impressive to you?
Miyahara: A competition that stands out in memory is a recommendation competition, called OTTO – Multi-Objective Recommender System, where you predict the user’s next action (click, cart, or order) based on the user action log on an EC website. This system deals with only a few types of data – timestamp, user_id, item_id, action_type – but you have to handle an enormous volume of data made up of 1.8 million items and 220 million log records for 12 million users. This competition is not for someone who notices something before others. It requires you to increase accuracy little by little by piling up ideas. It gave me fun equivalent to that of a life simulation game.
Miyatani: I participated in many different competitions, and I remember tackling problems by teaming up with engineers outside Sony and communicating with them day and night. While we were working on the same problem, I learned about the other team members’ way of working and thinking, which I could not have known if I worked only inside Sony. That significantly broadened my horizons. To win competitions, I read a number of papers released at the leading AI-related academic conferences of the time that I thought might boost my score and repeated experiments. Working on these competitions gave me a sense of fulfillment similar to that you feel in your extracurricular activities. I still stay in contact with members of my team.
Kobayashi: The competition that is the most impressive to me is one called TensorFlow – Help Protect the Great Barrier Reef in which participants were tasked with detecting starfishes from underwater video imagery. It was about a global environmental issue of a massive starfish outbreak impacting coral reefs in the Great Barrier Reef of Australia and called for detecting starfishes and counting their number. I found it worth doing because I might be able to contribute to solving the real environmental issue. The competition stays in my memory also because it made me realize anew an engineer’s social role of making the world a better place through the power of AI.
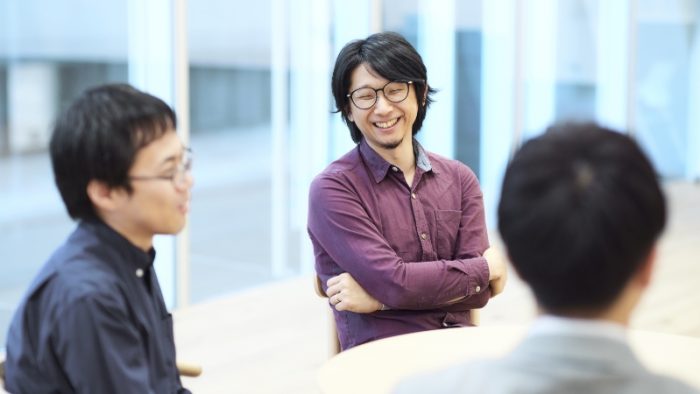
More trial and error translates into greater strength as an engineer
– Working on Kaggle, what did you experience and learn? And, tell us how it contributes to your current job?
Kobayashi: A majority of Sony’s projects deal with something that no one has ever done in the world. I participated in many high-level Kaggle competitions and found out my own solutions while competing with talented rivals. This experience has given me enough confidence and skill to convince myself that I can find a solution to any problem if I don’t give up on thinking. For example, when I face a new challenge in my project, I can find a path forward for the project, based on my experience at Kaggle, figuring out what the whole framework should look like, how machine learning models are trained, and what kinds of indicators should be used for evaluation. I feel that I have gained the ability to overcome unknown problems.
Miyatani: I took part in various Kaggle competitions too and gained experience. That made me able to predict results of data analysis, which helps me a lot in my current management work. In actual AI development projects, it often happens that, although you have data, you never know if your project will succeed or not unless you give it a try. I can judge to a certain degree whether a project will succeed or not, just by looking at the data. Also, when I check on the progress of a project, I can encourage the project members to aim for better results by saying something like: “You should be able to get even higher accuracy in this case.”
When working on Kaggle, I put great importance on the initial phase of Exploratory Data Analysis (EDA). I think that EDA is essential in work as well. Instead of creating a model set quickly using the data in front of you, it is important to fully understand the evaluation indicators, know the data by looking at it closely for a long enough time, and build an appropriate validation *3 process. I believe that such careful preparations in the initial phase provide a basis for more accurate data analysis.
*3 A technique to evaluate the performance of a machine learning model
Miyahara: I also think that how you see and build the validation process matters the most. In actual development projects, you sometimes encounter a serious problem where accuracy turns out to be much lower than the required level in the production environment although a high level of accuracy was observed in an advance evaluation. Validation prevents this problem. In the case of a Kaggle competition, you lament your bad luck of not winning a medal and then move on. In business, however, the problem could lead to a huge loss. Through the past Kaggle competitions, I learned how to build the validation process despite repeated failures and got the knack of increasing accuracy. I think that’s a precious experience for me.
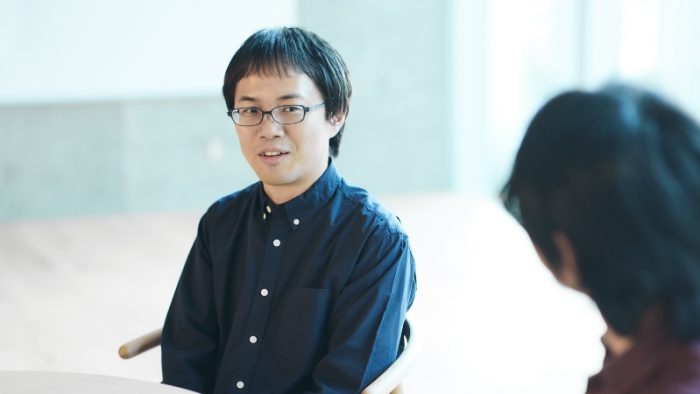
Create new values by exploiting Sony’s vast range of business domains
– Using what you learned at Kaggle and the skills you gained there, how are you going to contribute to Sony in the field of AI?
Kobayashi: Sony does business in a vast range of domains, including electronics, entertainment, semiconductors, and financial services, and each of its group companies is now developing and introducing AI technology. I think that, if the group companies share the knowledge and know-how they have earned in these domains across corporate boundaries, we will be able to continue to provide a new user experience that only the Sony Group can deliver. I hope to contribute to the creation of such experiences by utilizing the experiences and skills that I acquired through the various Kaggle competitions.
Miyahara: I also see the breadth of the Sony Group’s business domains as its unrivaled appeal. For example, take Prediction One, which I am in charge of. We have had the group companies use this tool and provide feedback. By making improvements repeatedly, we have increased prediction accuracy and created new experiences for tool users.
Miyatani: These days, major IT firms and many other companies are developing machine learning models and analyzing data. Under the circumstances, I think that Sony has a strength in data analysis inputs and outputs. For inputs, we have diverse data obtained from the wide-ranging business domains. In addition, we have a semiconductor business and can create new sensing devices. This means that we can get sensing data best suited to data analysis. Our outputs have many potential uses, too. For example, the data obtained from TVs could be used for developing insurance products. I am now developing AI technology for new sensing image sensors, and I always keep the big picture of the Sony Group in mind.
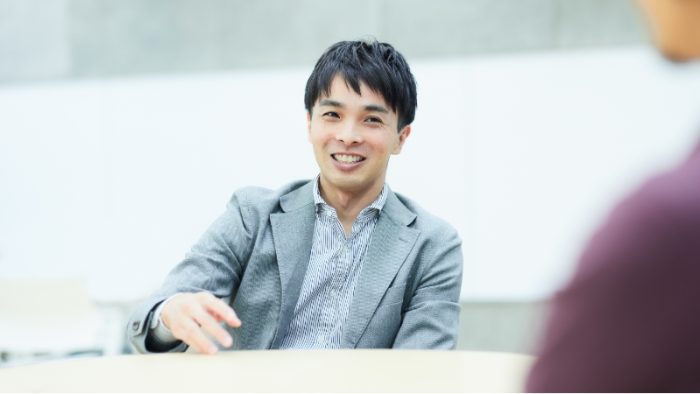
– Lastly, could we have your messages to the readers, including Kagglers?
Miyatani: In the semiconductor business domain in which I work, it is increasingly important to explore new applications of sensing image sensors. We need to repeat the PDCA cycle quickly and accelerate the decision-making process. As for me, it is no exaggeration to say that I acquired all the skills necessary to do so at Kaggle. I hope that Kagglers become interested in Sony’s semiconductor business domain.
Miyahara: At Sony’s research and development departments, not only do you research and develop leading-edge technologies, but you also bring those technologies to market, get user feedback, and repeat improvements in a down-to-earth manner. It is a place where you can do this all. The Sony Group has experts in all the fields, and various kinds of data obtained from its wide-ranging business domains are available. There are also plenty of opportunities to utilize the skills you obtain at Kaggle.
Kobayashi: I think Sony is the perfect place for those who are willing to take on new challenges and learn from each other. It’s great to have peers who are interested not just in their field of expertise but in other fields as well and committed to solving issues. I want to tackle unknown problems, learning and growing together with those peers. I believe that, by doing so, we will be able to contribute to solving social issues and to the world.